Goal-Conditioned Action Space Reduction for Deformable Object Manipulation
Shengyin Wang, Rafael Papallas, Matteo Leonetti and Mehmet Dogar
IEEE International Conference on Robotics and Automation (ICRA), 2023, London, UK
Abstract
Planning for deformable object manipulation has been a challenge for a long time in robotics due to its high computational cost. In this work, we propose to reduce this cost by reducing the number of pick points on a deformable object in the action space. We do this by identifying a small number of key particles that are sufficient as pick points to reach a given goal state. We find these key particles through a geometric model simplification process, which finds the minimal geometric model that still enables a good approximation of the original model at the goal state. We present an implementation of this general approach for 1-D linear deformable objects (e.g., ropes) that uses a piece-wise line fitted model, and for 2-D flat deformable objects (e.g., cloth) that uses a mesh simplified model. We conducted simulation experiments on ropes and cloths, which demonstrate the effectiveness of the proposed method. Finally, the planned paths are executed in a real-world setting for two cloth folding tasks.
Video
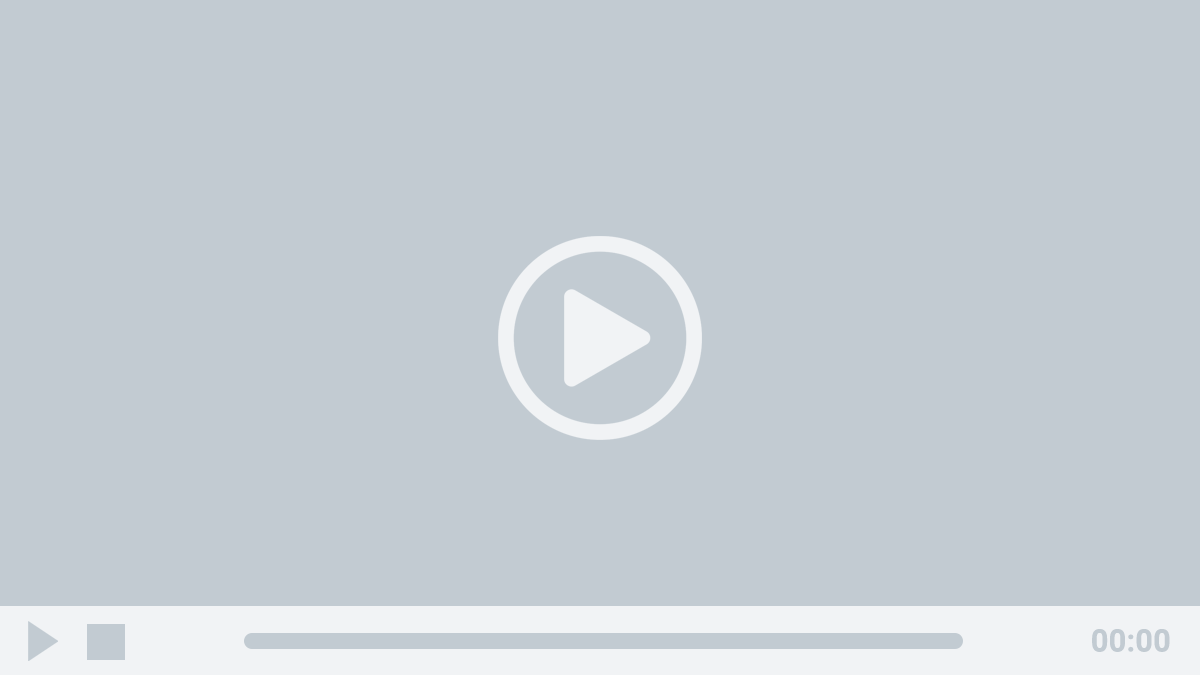
Citing
If you have any questions, please feel free to drop a line. Finally, if you want to cite this work, please use the following:
@inproceedings{wang2023goal,
title={Goal-conditioned action space reduction for deformable object manipulation},
author={Wang, Shengyin and Papallas, Rafael and Leonetti, Matteo and Dogar, Mehmet},
booktitle={2023 IEEE International Conference on Robotics and Automation (ICRA)},
pages={3623--3630},
year={2023},
organization={IEEE}
}